5 Ways to Improve Data Quality in Insurance Submissions
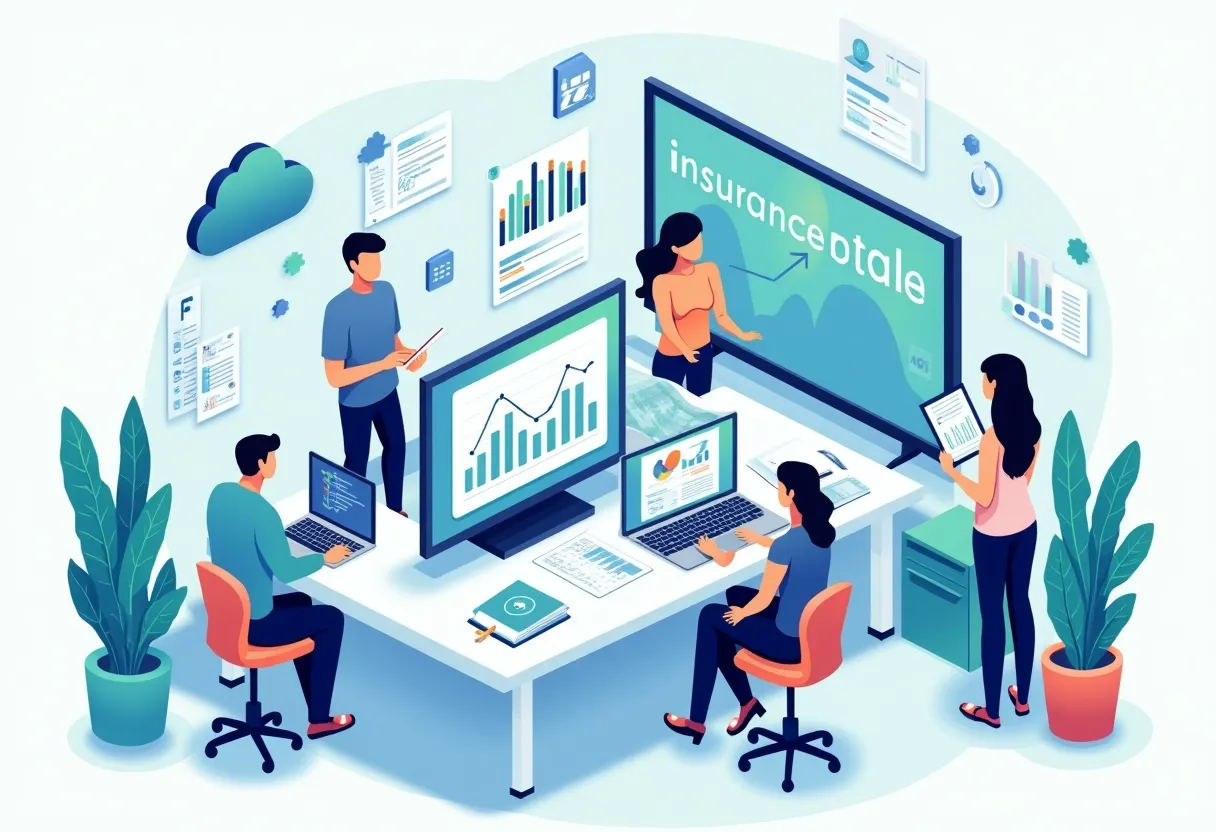
Introduction
In the world of insurance, data quality is paramount, particularly concerning submission processes. Submissions are the lifeblood of insurance operations, serving as a critical entry point for policies, claims, and customer interactions. If the data submitted is inaccurate, incomplete, or inconsistent, it can lead to significant issues downstream, including inefficiencies, higher operational costs, and increased risk exposure.
Common challenges in submission workflows can include poor data entry practices, lack of standardized formats, and inadequate validation mechanisms. These challenges highlight the necessity for insurers to implement robust data management strategies that enhance data quality. Streamlined strategies can significantly increase operational efficiency, reduce rework, and ultimately lead to better service offerings and improved customer satisfaction.
How Can Streamlined Data Collection Processes Enhance Quality?
Streamlined data collection processes are essential for improving the quality of data in insurance submissions. When insurers systematically gather data, they reduce the risks of errors and inaccuracies that may arise from haphazard collection methods.
What are the best practices for data collection?
Implementing best practices for data collection can markedly improve submission quality. This includes employing clear guidelines for information gathering, utilizing user-friendly forms, and setting expectations for the data required at the outset. Additionally, offering training for staff on these practices ensures consistent adherence across teams.
How can automation minimize human errors?
Automation plays a transformative role in reducing human errors during the data collection phase. By automating data entry processes, insurers can eliminate the tedious manual tasks that often lead to mistakes. For instance, optical character recognition (OCR) and data capture technologies can be utilized to extract information directly from documents, minimizing the chances of errors linked to manual input.
Why is standardizing data formats important?
Standardizing data formats is crucial for maintaining data integrity. Uniform formats across submissions ensure that information is easily interpretable and negligible discrepancies arise due to variations in structure. When all parties utilize a common format, it becomes much simpler to aggregate, analyze, and report data, leading to more accurate insights and effective decision-making.
What Role Does Data Validation Play in Improving Accuracy?
Data validation is vital in elevating submission accuracy. By verifying the accuracy and completeness of data before acceptance, insurers can dramatically reduce the number of errors that manifest downstream in the process.
What types of validations should insurers implement?
Insurers should look to implement several types of validations in their submission workflows. These include format checks, consistency checks, and completeness checks, among others. Format checks ensure that entries, such as dates or policy numbers, adhere to predefined structures, while consistency checks ascertain that multiple data points correlate correctly. Completeness checks guarantee that all necessary information has been filled out, preventing incomplete submissions.
How do real-time validations prevent errors in submissions?
Real-time validations instantly verify data as it is entered, alerting users to mistakes or inconsistencies before submission. This immediate feedback loop plays a crucial role in enhancing data quality by preventing errors from progressing further into the workflow. Real-time validation can include live error messages when a user attempts to submit incorrect data, fostering a more error-free submission process.
What technologies can facilitate effective data validation?
Integrating modern technologies such as artificial intelligence and machine learning can significantly enhance data validation efforts. Advanced algorithms can continuously learn from historical data to identify patterns associated with errors, improving predictive accuracy and proactive measures over time. Moreover, employing data validation software or tools can streamline validation processes, ensuring consistent and reliable checks across various submission types.
How Does Staff Training Impact Submission Data Quality?
The competency of staff directly influences the quality of submission data. Continuous training ensures that employees are well-informed about best practices, industry standards, and the importance of accuracy in data handling.
Why is ongoing training essential in the insurance sector?
Ongoing training is essential in the insurance sector for several reasons. Insurance is a constantly evolving field, with frequent regulatory changes, technology advancements, and new risk factors emerging. Regularly updating employees on these changes helps them understand their relevance, fostering a culture of compliance and quality assurance.
What specific areas should training programs focus on?
Training programs should focus on areas critical to data quality, including data entry techniques, the importance of adherence to standard formats, and the implications of data inaccuracies. Additionally, training should emphasize using any associated systems or software designed to facilitate accurate data collection and submission processes.
How can technology aid in staff training and skill enhancement?
Technology can significantly enhance staff training through e-learning platforms, simulation tools, and interactive workshops. Virtual training programs allow employees to learn at their own pace and revisit training materials as needed. Utilizing software that simulates real-world data entry scenarios can help staffs hone their skills in a risk-free environment, thereby improving confidence and competence in their roles.
How Important Is Collaboration Between Teams in Managing Data?
Collaboration among departments is essential for effective data management in insurance submissions. Multiple teams often handle different segments of the submission process, and their seamless interaction is critical for maintaining data quality.
What benefits does cross-functional collaboration offer?
Cross-functional collaboration can yield numerous benefits, including enhanced communication, shared insights, and a unified approach to data management. When various teams, such as underwriting, claims processing, and IT, work together, they can address data quality issues more comprehensively, leading to higher overall submission accuracy.
How can teams share insights to improve data management practices?
Teams can share insights through regular meetings, collaborative platforms, or shared dashboards that track data quality metrics. By encouraging open communication and feedback loops, organizations can uncover and address common bottlenecks or errors in submissions, improving overall efficiency and data management practices.
What tools facilitate better inter-departmental communication?
Utilizing tools like collaborative project management software, communication platforms, and integrated data systems can significantly improve inter-departmental communication. These technologies enable teams to share documents, track projects, and manage workflows in real-time, ensuring that the right information is accessible to the right people at the right time.
How Can Insurers Leverage AI for Enhanced Data Management?
Artificial intelligence is reshaping the way insurers manage data by enhancing data quality and submission processes. By automating data management tasks, AI can not only improve efficiency but also ensure higher data accuracy.
What specific AI applications can improve data accuracy?
AI applications such as predictive analytics and natural language processing (NLP) are particularly useful in enhancing data accuracy. Predictive analytics helps anticipate data discrepancies based on historical trends, allowing teams to address potential issues proactively. NLP can assist in deciphering unstructured data, such as comments or notes, and categorizing them accordingly, thereby improving data integrity.
How does machine learning refine data submission processes?
Machine learning algorithms refine data submission processes by continuously learning from previous submissions and identifying patterns that lead to inaccuracies. As these algorithms are exposed to more data, they become more adept at predicting data inconsistencies, making real-time recommendations for correction and improving the overall submission quality.
What are the future trends of AI in insurance data management?
Looking ahead, the integration of AI in insurance data management is poised to expand significantly. Future trends may include enhanced predictive modeling capabilities, wider adoption of automated systems, and increased use of chatbots for real-time customer engagement during the submission process. As technology continues to evolve, insurers must be vigilant in adapting to these changes to stay competitive in the marketplace.
Conclusion
In conclusion, prioritizing data quality in insurance submissions is vital for operational efficiency and risk management. By focusing on streamlined data collection processes, data validation mechanisms, staff training, inter-departmental collaboration, and leveraging AI, insurers can significantly enhance their data quality. Each of these pathways presents unique opportunities to drive accuracy and improve submission workflows.
For organizations seeking to further explore the implications of AI in submission processes, we encourage you to check out our related blog on AI-Powered Submission Triage: Prioritize What Matters Most. If you're ready to enhance your data management practices, contact us today.